热点复现|用Dash做A股量化策略可视
近几个月来,用Dash做各种数据展示的案例频繁出现在公众号上,不禁想把业余做的A股量化策略研究也做个展示。
1. 数据获取
A股数据可以通过tushare获取。
1.1. 安装tushare包:
!pip install tushare
1.2. 导入tushare包,并初始化
import tushare as ts
pro = ts.pro_api('your token')
token可以通过注册tushare账号获取。
1.3. 获取行情数据
日线行情数据可以通过daily接口获取。
daily = pro.daily()
daily
由于这两句代码在ModelArts平台上未能通过调试,实现将在jupyter上进行。调试失败信息是“JSONDecodeError: Expecting ',' delimiter: line 1 column 86204 (char 86203)”,还请ModelArts的专家能关注一下。
1.4. 获取财务数据
利润表数据可以通过income接口获取。
daily = pro.income()
daily
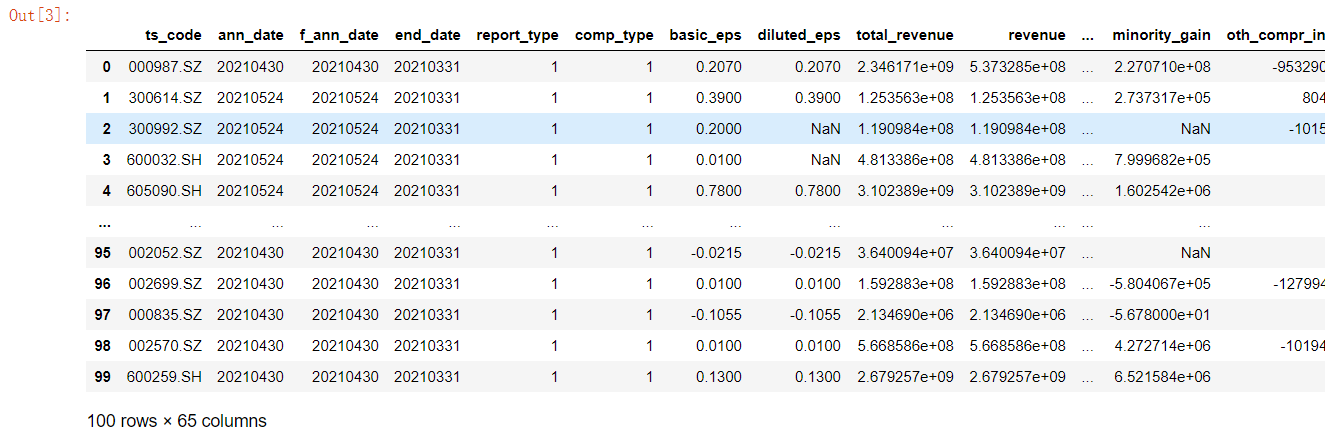
1.5. 获取其他数据
其他数据可以根据策略需要通过不同接口获取,具体接口与参数可以到tushare管网查询。
2. 量化策略
量化策略的关键是决定在什么情况下买,什么情况下卖?是拍脑袋决定,还是通过数据和模型来决定。
简单起见,我们拍脑袋决定一个简单的量化策略,当然这不太可能是一个成功的策略。
2.1. 基本设定
假定其实金额100万元人民币,每个交易日以开盘价买入上一交易日成交额最大的股票,三天后卖出。买入数量为不超过且尽可能逼近当前总资产的1/4,总资产的计算不考虑浮动盈亏。回测期间为2020年1月1日-2020年12月31日。
start_amount = 1000000
2.2. 获取交易日历
trade_date = pro.trade_cal(start_date=20200101, end_date=20201231, is_open=1)
trade_date
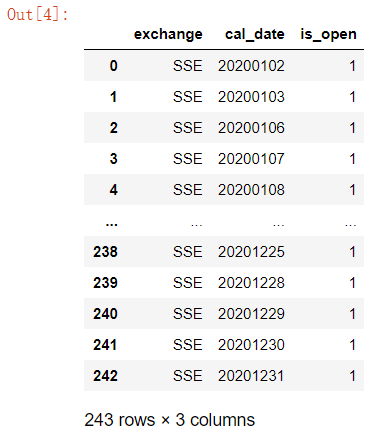
2.3. 初始化
回测第一天不发生交易,为了方便,我们把它计为第0天。交易日期如下:
current_date = trade_date.loc[0, 'cal_date']
current_date
总资产为初始总资产,货币资金为总资产
amount = {}
currency_amount = {}
amount[current_date] = currency_amount[current_date] = start_amount
初始化交易数据
import pandas as pd
trade_data = pd.DataFrame(columns=['ts_code', 'buy_date', 'buy_price', 'buy_num', 'hold_days', 'sale_date', 'sale_price'])
trade_data

2.4. 第一天买卖
记录上一交易日
pre_date = current_date
pre_date
获得当前交易日
current_date = trade_date.loc[1, 'cal_date']
current_date
获得上一交易日的成交量信息
pre_daily = pro.daily(trade_date = pre_date, fields='ts_code, amount')
pre_daily
获取上一交易日成交量最大的股票。
ts_code = pre_daily[pre_daily['amount']==pre_daily.amount.max()].iloc[0, 0]
ts_code
获取本日开盘价
buy_price = pro.daily(trade_date = current_date, ts_code = ts_code).iloc[0, 2]
buy_price
计算买入数量
buy_amount_limit = min(amount[pre_date] / 4, currency_amount[pre_date])
buy_num = buy_amount_limit // (buy_price * 100)
buy_num
写入交易数据
trade_data.loc[len(trade_data),['ts_code', 'buy_date', 'buy_price', 'buy_num', 'hold_days', 'sale_price']] = [ts_code, current_date, buy_price, buy_num, 0, 0]
trade_data
改写货币资金和总资产
currency_amount[current_date] = currency_amount[pre_date] - buy_price * buy_num * 100
amount[current_date] = amount[pre_date]
currency_amount

2.5. 第二天买卖
记录上一交易日,获得当前交易日
pre_date = current_date
current_date = trade_date.loc[2, 'cal_date']
第一天买入的股票持有天数加1
trade_data.loc[trade_data['hold_days']<3, 'hold_days'] += 1
trade_data
重复第一天的买入过程
pre_daily = pro.daily(trade_date = pre_date, fields='ts_code, amount')
ts_code = pre_daily[pre_daily['amount']==pre_daily.amount.max()].iloc[0, 0]
buy_price = pro.daily(trade_date = current_date, ts_code = ts_code).iloc[0, 2]
buy_amount_limit = min(amount[pre_date] / 4, currency_amount[pre_date])
print(buy_amount_limit)
buy_num = buy_amount_limit // (buy_price * 100)
trade_data.loc[len(trade_data),['ts_code', 'buy_date', 'buy_price', 'buy_num', 'hold_days', 'sale_price']] = [ts_code, current_date, buy_price, buy_num, 0, 0]
trade_data
改写货币资金和总资产
currency_amount[current_date] = currency_amount[pre_date] - buy_price * buy_num * 100
amount[current_date] = amount[pre_date]
currency_amount

2.6. 第三天买卖
重复第二天的操作
pre_date = current_date
current_date = trade_date.loc[3, 'cal_date']
trade_data.loc[trade_data['hold_days']<3, 'hold_days'] += 1
pre_daily = pro.daily(trade_date = pre_date, fields='ts_code, amount')
ts_code = pre_daily[pre_daily['amount']==pre_daily.amount.max()].iloc[0, 0]
buy_price = pro.daily(trade_date = current_date, ts_code = ts_code).iloc[0, 2]
buy_amount_limit = min(amount[pre_date] / 4, currency_amount[pre_date])
buy_num = buy_amount_limit // (buy_price * 100)
trade_data.loc[len(trade_data),['ts_code', 'buy_date', 'buy_price', 'buy_num', 'hold_days', 'sale_price']] = [ts_code, current_date, buy_price, buy_num, 0, 0]
currency_amount[current_date] = currency_amount[pre_date] - buy_price * buy_num * 100
amount[current_date] = amount[pre_date]
trade_data
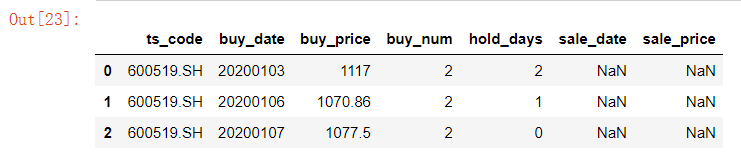
2.7. 第四天买卖
重复第三天的操作
pre_date = current_date
current_date = trade_date.loc[4, 'cal_date']
trade_data.loc[trade_data['hold_days']<3, 'hold_days'] += 1
pre_daily = pro.daily(trade_date = pre_date, fields='ts_code, amount')
ts_code = pre_daily[pre_daily['amount']==pre_daily.amount.max()].iloc[0, 0]
buy_price = pro.daily(trade_date = current_date, ts_code = ts_code).iloc[0, 2]
buy_amount_limit = min(amount[pre_date] / 4, currency_amount[pre_date])
buy_num = buy_amount_limit // (buy_price * 100)
trade_data.loc[len(trade_data),['ts_code', 'buy_date', 'buy_price', 'buy_num', 'hold_days', 'sale_price']] = [ts_code, current_date, buy_price, buy_num, 0, 0]
currency_amount[current_date] = currency_amount[pre_date] - buy_price * buy_num * 100
amount[current_date] = amount[pre_date]
trade_data
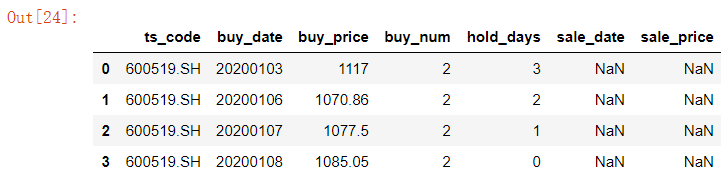
2.8. 第五天买卖
记录上一个交易日并获取当前交易日
pre_date = current_date
current_date = trade_date.loc[5, 'cal_date']
找到持有达到3天的股票以及持有数量
trade_index = ((trade_data['hold_days']==3) & (trade_data['sale_price']==0))
ts_code = trade_data.loc[trade_index, 'ts_code'][0]
sale_num = trade_data.loc[trade_index, 'buy_num'][0]
orignal_buy_price = trade_data.loc[trade_index, 'buy_price'][0]
ts_code, sale_num
获取当天收盘价
sale_price = pro.daily(trade_date = current_date, ts_code = ts_code).loc[0, 'close']
sale_price
写入交易数据
trade_data.loc[trade_index, ['sale_date', 'sale_price']] = [current_date, sale_price]
trade_data
重复上一天的操作
trade_data.loc[trade_data['hold_days']<3, 'hold_days'] += 1
pre_daily = pro.daily(trade_date = pre_date, fields='ts_code, amount')
ts_code = pre_daily[pre_daily['amount']==pre_daily.amount.max()].iloc[0, 0]
buy_price = pro.daily(trade_date = current_date, ts_code = ts_code).iloc[0, 2]
buy_amount_limit = min(amount[pre_date] / 4, currency_amount[pre_date])
buy_num = buy_amount_limit // (buy_price * 100)
trade_data.loc[len(trade_data),['ts_code', 'buy_date', 'buy_price', 'buy_num', 'hold_days', 'sale_price']] = [ts_code, current_date, buy_price, buy_num, 0, 0]
trade_data
改写货币资金和总资产
currency_amount[current_date] = currency_amount[pre_date] - buy_price * buy_num * 100 + sale_num * sale_price * 100
amount[current_date] = amount[pre_date] + (sale_price - orignal_buy_price) * sale_num * 100
currency_amount, amount
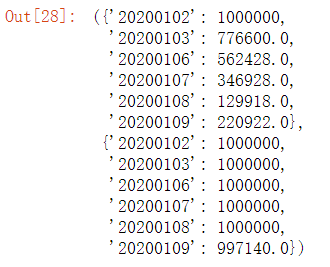
2.9. 完整代码
易见,第1天-第4天是第5天的特殊情况。本策略的完整代码如下:
import pandas as pd
import tushare as ts
pro = ts.pro_api('your token')
trade_date = pro.trade_cal(start_date=20200101, end_date=20201231, is_open=1)
amount = {}
currency_amount = {}
trade_data = pd.DataFrame(columns=['ts_code', 'buy_date', 'buy_price', 'buy_num', 'hold_days', 'sale_date', 'sale_price'])
pre_date = trade_date.loc[0, 'cal_date']
amount[pre_date] = currency_amount[pre_date] = 1000000
for current_date in trade_date.loc[1:, 'cal_date']:
trade_index = ((trade_data['hold_days']==3) & (trade_data['sale_price']==0))
sale_num = sale_price = orignal_buy_price = 0
if len(trade_index) and max(trade_index):
ts_code = list(trade_data.loc[trade_index, 'ts_code'])[0]
sale_num = list(trade_data.loc[trade_index, 'buy_num'])[0]
orignal_buy_price = list(trade_data.loc[trade_index, 'buy_price'])[0]
sale_price = pro.daily(trade_date = current_date, ts_code = ts_code).loc[0, 'close']
trade_data.loc[trade_index, ['sale_date', 'sale_price']] = [current_date, sale_price]
trade_data.loc[trade_data['hold_days']<3, 'hold_days'] += 1
pre_daily = pro.daily(trade_date = pre_date, fields='ts_code, amount')
ts_code = pre_daily[pre_daily['amount']==pre_daily.amount.max()].iloc[0, 0]
buy_price = pro.daily(trade_date = current_date, ts_code = ts_code).iloc[0, 2]
buy_amount_limit = min(amount[pre_date] / 4, currency_amount[pre_date])
buy_num = buy_amount_limit // (buy_price * 100)
trade_data.loc[len(trade_data),['ts_code', 'buy_date', 'buy_price', 'buy_num', 'hold_days', 'sale_price']] = [ts_code, current_date, buy_price, buy_num, 0, 0]
currency_amount[current_date] = currency_amount[pre_date] - buy_price * buy_num * 100 + sale_num * sale_price * 100
amount[current_date] = amount[pre_date] + (sale_price - orignal_buy_price) * sale_num * 100
trade_data
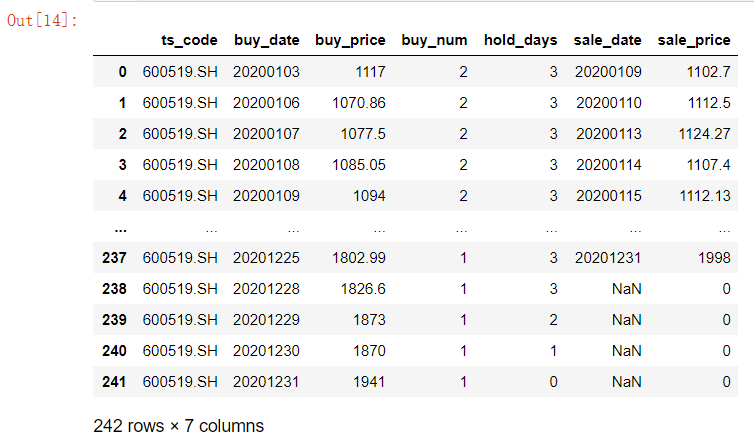
3. Dash可视
安装Dash和Dash的html组件和核心组件
!pip install dash
!pip install dash_html_components
!pip install dash_core_components
先来显示个标题
import dash
import dash_html_components as html
app = dash.Dash()
app.layout = html.Div([
html.H2(children='一个量化策略',
style = dict(backgroundColor = '#111111', textAlign = 'center', color = '#7FDBFF')),
])
if __name__ == '__main__':
app.run_server(host="0.0.0.0")
运行后打开浏览器,访问http://localhost:8050/,可以看到 显示前面量化策略的效果
import dash, datetime
import dash_html_components as html
import dash_core_components as dcc
def numtodate(n):
return datetime.datetime.strptime(str(n), '%Y%m%d')
app = dash.Dash()
app.layout = html.Div([
html.H2(children='一个量化策略',
style = dict(backgroundColor = '#111111', textAlign = 'center', color = '#7FDBFF')),
dcc.Graph(figure={
'data':[
{
'x': [numtodate(k) for k in amount.keys()],
'y': [int(v) for k, v in amount.items()]
},
]
}),
])
if __name__ == '__main__':
app.run_server(host="0.0.0.0")
可以看到,随便拍个策略果然赚不到钱。
4.总结
我们演示的量化策略并不成功。即使这样,它还有很多未考虑全面的地方,包括买卖手续费怎么计算?如果买入当天开盘涨停怎么办?如果卖出当天跌停无法成交怎么办?
如果我们要对策略进行优化和更有效的测试,那么还需要考虑我们的回测周期是不是应该穿越牛熊?还是说我们的策略就是为了捕捉短期因子?
在越来越多机构进入量化领域的今天,散户玩A股量化基本上不存在暴利的机会,但寻找一些短期盈利的策略还是有可能的。我在http://www.gchatst.club:8050/展示了一些看着貌似能盈利的策略,有兴趣的小伙伴可以看一看。当然,我只回测了最近一年,我也只能是尽可能的消除未来函数。投资有风险。。。。哈哈